Generative AI for E-Commerce: Ensuring AI Chatbot Response Reliability
iAdvize
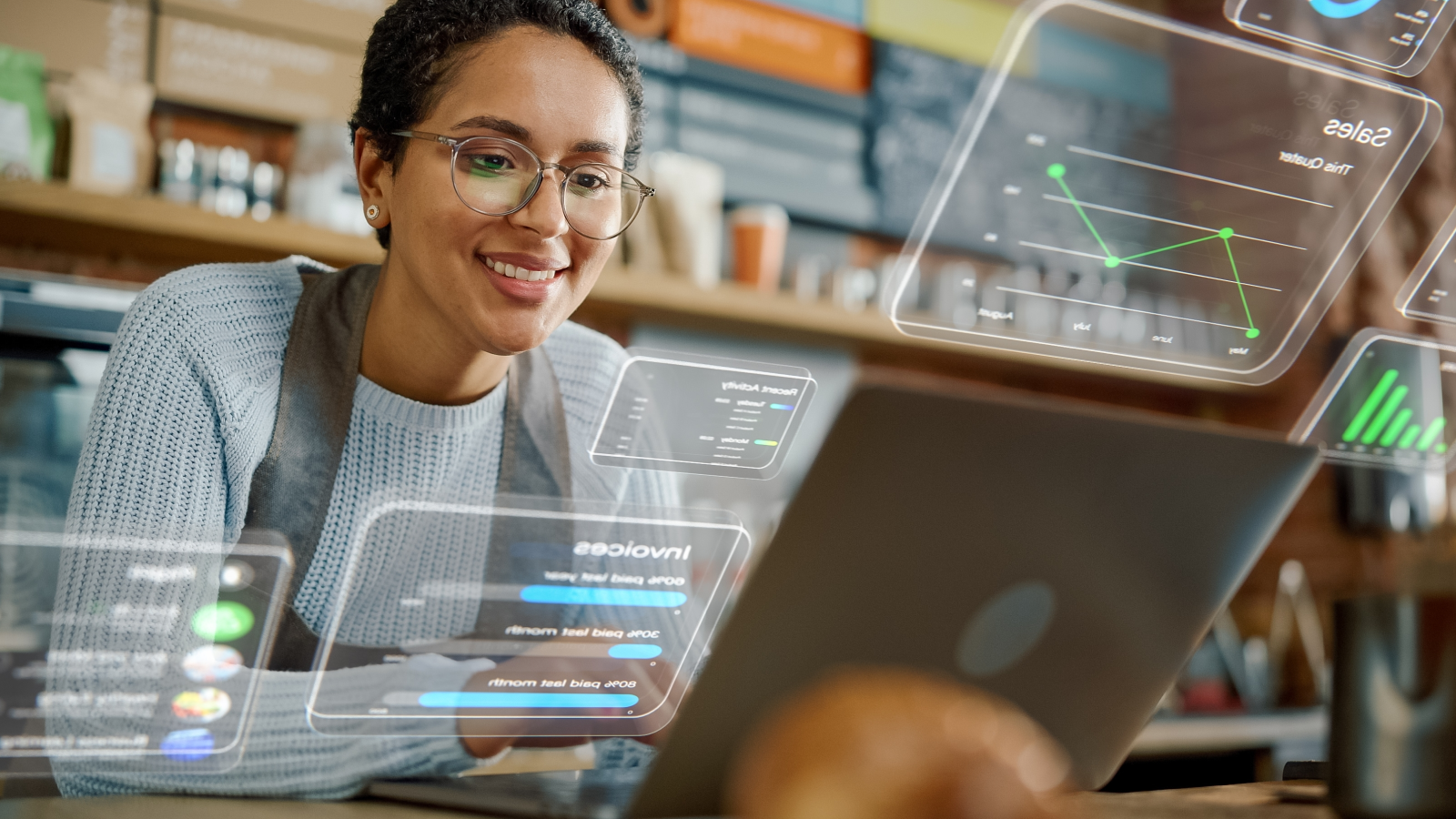
Millions of people worldwide now use AI to perform daily tasks. While it doesn't and shouldn’t fully replace humans, this technology undoubtedly transforms and enhances our way of working. Faced with this new norm, it's necessary to establish rules that ensure the protection of personal data. However, this isn’t the only major challenge that users will face with generative AI. It’s also important to ask how we can ensure an AI’s information output is factually accurate. In this article, we’ll discuss the challenges of using this technology in e-commerce, and offer guidance on how to implement a system that will create better response reliability.
Conversational AI Chatbot Challenges: Generic Responses, Hallucinations & Control Issues
Due to recent advancements in generative AI technology, large language models (LLM) are now capable of interpreting and generating natural language content. In other words, you can prompt an AI chatbot to respond by text, sound, image, or video in any language you choose. This technology, although impressive, raises questions like: How far can you take this? Will it eventually replace humans in the workplace? Can we ever fully trust AI?
Consumer applications like ChatGPT, Google Bard, or MidJourney are admittedly astonishing in what they can do and open up a world of new possibilities in terms of content production and productivity. However, despite their immense potential, it's crucial to recognize the limitations for a business setting.
"Generative AIs are generic and global by nature, being trained on an enormous amount of common knowledge data, whereas brands need to provide a company-specific customer experience (processes and regulations, products and services, legal constraints, etc.) as well as communicate in a way that aligns with their core values, like brand identity and tone or ethical considerations."
– Florent Gosselin, CPO at iAdvize
One of the biggest concerns surrounding online AI chat solutions are that they tend to provide responses that are too generic. Fortunately, it's possible to recalibrate the technology so that it better adapts to a brand's specific identity, tone, and rules. Some of the other challenges include:
Hallucinations
Due to its creative nature, generative artificial intelligence tends to improvise in the absence of precise answers or when faced with ambiguous direction. This can lead to biased responses or ‘hallucinations’ of information that are inaccurate or entirely made up. It’s important that brands implement appropriate protective measures to safeguard against the potential for these kinds of responses and promote a more reliable experience for their customers.
Control
Generative AI often appears as a black hole to its users, meaning that businesses should be prioritizing transparency and control. It's crucial for companies to implement monitoring mechanisms that constantly assess the impact of generative AI on their operations and use these insights to optimize and improve its functionality and behavior.
Trustworthy Generative AI for E-Commerce: Myth or Reality?
You might have first discovered generative AI while testing versions 3.5 or 4 of ChatGPT, the AI created by OpenAI L.L.C. This consumer-facing application proves incredibly useful for a variety of different professional fields, like writing and project management. However, for e-commerce, reliability remains a prominent concern. So, how can you harness the immense potential of GPT for online commerce? To be both effective and dependable, generative AI for e-commerce must be built on models designed specifically for businesses and made entirely distinct from public models like ChatGPT. Microsoft Azure OpenAI is a good example of this. The solution should allow for the implementation of safeguards and monitoring to gauge the business impact of the AI and optimize it in real time.
How to Prevent Your AI Chatbot from ‘Inventing’ Responses
When it struggles to find an answer to a given question, generative AI has a tendency to fill in the blanks by producing incorrect responses. OpenAI had to program ChatGPT to specify the date when the shared information was last accessed. Prior to this, it wasn't uncommon for the obtained information to be outdated, since the AI only had access to data up until 2021.
This raises concerns among brands and retailers, especially around the spread of fake news. Imagine a customer looking for information about the latest smartphone, only to receive details about last year's model or a price that no longer matches the current offer. This is a surefire way to disappoint consumers and erode their trust. Brands cannot afford to provide outdated information about their products.
Then how is this issue solved? First, it's important to note that these inaccuracies depend on the "temperature" of the AI model. This is defined in the prompt that’s given to the AI software and will determine its level of creativity in producing a response. A setting of 1 will generate a much more creative answer than a 0—basically the difference between responding in a Shakespearean-style prose versus robotic-sounding jargon.
The challenge is to strike the right balance and be able to provide users with natural responses while preventing the AI from making mistakes. A reliable AI needs just enough creativity to engage in conversations with your customers, while adhering to your rules to ensure the accuracy of the information it yields.
Techniques for Controlling Generative AI & Getting the Best Results
It all starts with the prompt. If you've been following the news for the last few months, this concept is probably familiar to you. The prompt is the set of instructions given to an AI in order to obtain a desired response. On a large scale, prompting is the technological ingredient that makes it possible to build a trusted chatbot with AI that meets retailers' challenges.
This is why it’s essential to include a large number of instructions and rules in an AI model. These rules are what allow us to expect precise responses from the AI. With this set-up, a model can be instructed to do things like respond only to questions about a specific product, provide answers under 200 characters, or never answer questions about competing companies. These instructions, with additional parameters defined in your conversational platform, are safeguards that enable you to put generative AI to work for your bottom line, offering your customers effective, relevant support at all times. Brands that have already implemented AI shopping assistants powered by trusted generative AI see satisfaction rates of up to 70%, results that are well above the average for conventional chatbots.
AI Chatbot Information Sources
Brands can connect a generative AI chatbot platform directly to their existing tech ecosystem so that it pulls from the most updated, relevant information and amplifies its value over time. This looks like:
- Connecting the AI to website data, product catalogs, and knowledge bases
- Making the AI omnichannel—available on all messaging touchpoints used by customers via a conversational platform
- Giving the AI the ability to transfer interactions to human agents when necessary
To do this, the AI can be connected to data sources in a variety of ways, like through an API or web crawling. Trusted generative AI relies solely on the characteristics provided by the brand. If it doesn't have the answer, it transfers the conversation to a human respondent.
Ensuring That Generative AI Understands Customer Requests
Word embedding, or lexical embedding, is a method for enriching AI responses. Embedding converts text into mathematical vectors, making words, images, or even music interpretable by AI models. These vectors correspond to semantic categories that enable the AI to determine a relationship between two elements.
AI is able to match questions with their answers from a product sheet by measuring the distance between two vectors. For example, a catalog can be indexed according to the semantic vectors associated with each product. From there, a prompt is created to answer the question based on the closest product.
In the example above, the AI precisely identifies which phone model is the one in question. It will then extract the right information from the product sheet to provide the information the user is looking for.
How Cdiscount Ensures Reliability of its Generative AI Chatbot with iAdvize Copilot™
Cdiscount turned to generative AI with a key challenge in mind: facilitate connections between its 20 million unique monthly visitors and its 80 million products.
For this leading e-commerce marketplace with over 8 million active customers a year, it was vital to implement large-scale support. The objective? Respond quickly to all customer requests and expertly guide them through the online shopping process without compromising the quality of their customer service.
Strategies For Guaranteeing the Reliability of Answers From Generative AI
"We want to guarantee the reliability of our answers—it's something we owe to our customers."
– Antoine Pierart, Director of Marketing & Customer Experience at Cdiscount
To ensure that it provides accurate and relevant information, Cdiscount only feeds iAdvize's trusted generative AI with content from its product sheets. Every time a site visitor asks the bot a question, the reference of the page they’re on is integrated into the prompt, telling the AI chatbot to look for the information on that page.
Cdiscount eliminates any risk of answer inventions, otherwise known as hallucinations, because it programmed the bot with a crucial rule: if the AI is unable to answer a request, it must immediately transfer the conversation to a human agent.
The brand can also measure the impact of the generative AI on its sales performance using tracking tools—and since its launch, initial results have been very promising.
Results: Customer Satisfaction Thanks to Reliable Generative AI
Right away, the AI was able to handle 40% of conversations entirely autonomously, with the remainder taken by customer service agents through an escalation process. The conversion rate on conversations handled by the generative AI reached over 20%, similar to that observed for exchanges with a human respondent.
If consumer feedback is anything to go by, this technology is incredibly effective, recording a customer satisfaction rate 3x higher than that of decision-tree chatbots.